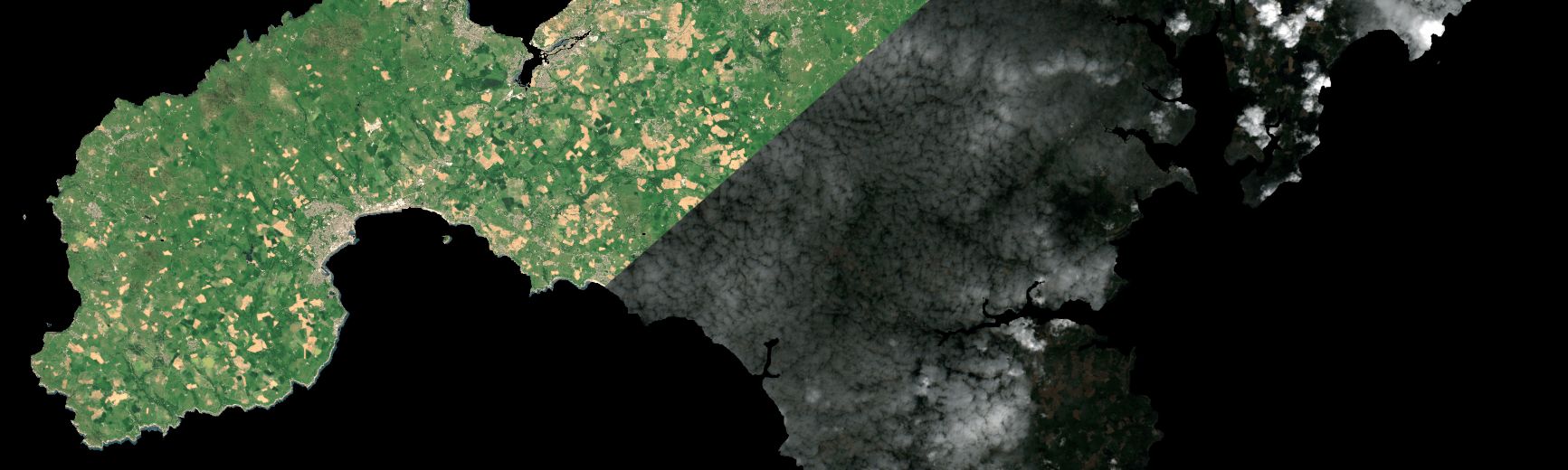
Our projects
-
Machine learning in Chemical Space
Lead: Professor Michael Schmuker
Chemical information is omnipresent in our everyday lives: from food ingredients and aroma, over plant and animal signals, to pharmaceutical drugs. Yet, “chemical space” has been notoriously difficult to tackle for data scientists. One great challenge lies in computational olfaction: To predict, in silico, whether a given molecule has a smell, and what that smell might be. In the DEEPFRAGRANCE project, we are applying cutting-edge machine learning methods to predict “fragrance-likeness” of a vast number of volatile compounds and their potential smell. This method can support fragrance and aroma chemists in discovering new and sustainable compounds for food and leisure products. Our previous efforts in this are dealt with elucidating the “olfactory code”, that is, how the nose achieves its remarkable ability to rapidly detects and identify odorants with high precision that is still unmatched by any artificial approach.
-
Neuromorphic Olfaction
Lead: Professor Michael Schmuker
Neuromorphic computing is an emerging technology that takes inspiration from the brain to achieve low-latency and low-power artificial intelligence. Key principles of neuromorphic computing are: a massively parallel architecture with lightweight compute units; communication via small, timed messages; and event-based signal representation and processing. Extensive potential gains in power efficiency and reduction in latency are expected from this technology compared to current methods in AI. The challenge is to develop novel algorithms that fully embrace the event-based sensing and processing paradigm, and thus unlock the full potential of event-based platforms such as the SpiNNaker neuromorphic hardware system. Dr Schmuker and his team developed an event-based electronic olfaction system that achieves low-latency detection of odorants. They pursue applications of this new technology in gas-based robotic navigation and agriculture.
-
Deep Earth Observation
Leads: Professor James Geach & Dr William Alston
We are innovating analysis techniques in Earth Observation (EO). Astrophysics is the ultimate remote sensing challenge. We figured that the problem of inferring the properties of distant galaxies from a limited set of (very) remote observations is no different to inferring the surface properties of the Earth using data taken from orbit. Complex data, weak signals and buried information is our bread and butter. So we are applying our experience in astrophysics-based hyperspectral image and time series analysis to EO, bringing to bear some of the latest AI techniques. We are particularly interested in applications of Synthetic Aperture Radar (SAR) observations, which are insensitive to cloud cover.
Our patented ClearSky deep learning algorithm, which can predict the full 400-2300nm Optical/Infrared ground response using SAR imaging alone, allows us to derive intelligence about ground conditions (such as the health or growth stage of crops) even in the presence of cloud. We are currently investigating novel applications of reverberation mapping, a technique used to probe the inner regions of active galactic nuclei powered by supermassive black holes, to investigate temporal trends in crop emergence. This is an important clue in understanding the impact of climate change on food production.
ClearSky is now licenced to Aspia Space Ltd., a member of NVIDIA’s Inception programme of innovative AI start-ups. Aspia Space is currently using ClearSky to monitor the status of hundreds of thousands of hectares of arable land in the UK.
-
Topological Pattern Detection in Climate Datasets
Lead: Dr John Evans
In this project we will innovate analysis techniques of large, high-dimensional climate datasets using techniques from topology, notably topological data analysis and persistent homology. As an initial goal, the project intends to tackle the problem of detecting extreme weather phenomena known as Atmospheric Rivers. These are narrow filaments of concentrated moisture in the atmosphere which have a central role in the global water cycle and have been linked with flooding on the West Coast of North America (California), Western Europe (UK), the west coast of North Africa, the Iberian Peninsula, Iran and New Zealand. In a rough sense, topology is the study of shape and so topological data analysis attempts to study the shape of data. It is the hope that in doing so, existing detection procedures can be made more robust. Longer term, there are several interesting problems on Climate Change for which topological data analysis can be applied, such as understanding the shape of climate attractors, the determination of flow patterns and the exploration of climate ‘tipping points’.
-
Narrative Structures of Mythological Epics
Lead: Dr John Evans
This project attempts a numerical examination of mythological epics such as the Iliad, Táin Bó Cuailnge, and the Mahabharata. At present, the main tools in this venture are from the realm of graph theory and so the first step is to construct a network whose nodes are characters in the epic, with edges arising from characters interacting. Once done, tools such as centrality measures allow us to quantify ideas such as the ‘importance’ or ‘prestige’ of certain characters (or groups of characters) within the narrative. We can explore ideas such as path length (famously conceptualised in the Six Degrees of Separation). In this way, we can gain a mathematical insight into how these social networks are constructed and what their key properties are. We can then compare these with other social networks, from the imaginary to very much real-world. In this way, there exists a spectrum and we can place a given mythological epic somewhere on this spectrum, understanding as we do the extent to which the given epic is based on real life. As has been seen in such examinations, sometimes the removal of a story-central character can make the resulting network ‘more real’, suggesting an historical basis for the myth. There are, of course, many more patterns within these narrative structures that can reveal interesting properties of the epics and a key goal in this project is to develop new tools which can help identify them.