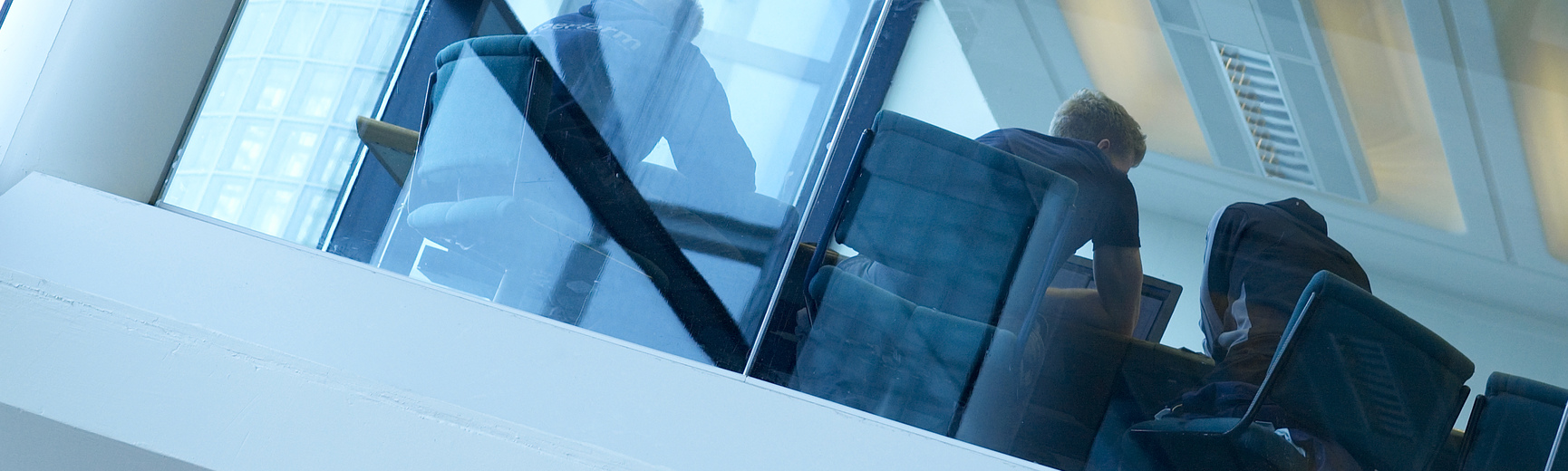
Our researchers
The six members of the unit are world leading scientists, publishing in major journals including Nature, Nature Astronomy, Nature Neuroscience, Proceedings of the National Academy of Sciences USA and Neuron. Collectively, the team have contributed to peer-reviewed publications that have accrued over 17,000 citations, and as PIs have secured a total of over £3M in funding to support their research over the past five years.
-
Professor James Geach (Director)
Geach conducts ground-breaking research into the evolution of galaxies (Geach et al. 2014, Nature, 516, 68; Geach & Peacock 2017, Nature Astronomy, 1, 795; Rupke, Coil, Geach et al. 2019, Nature, 574, 643) and has pioneered the use of unsupervised machine learning in astrophysics (Geach 2012, MNRAS, 419, 2633; Spindler, Geach & Smith 2021, MNRAS, 502, 985).
Jim is an astrophysicist specialising in galaxy evolution and observational cosmology, with an interest in cross-disciplinary and applied methods. With a degree in physics from Imperial College London and a PhD in astronomy from Durham University, Jim has nearly two decades of research experience at the forefront of his field, holding a Banting Fellowship at McGill University in Montreal and a Royal Society University Research Fellowship at the University of Hertfordshire, where he is a Professor of Astrophysics and Director of CoDIR. Jim has written two popular science books on galaxy formation and evolution, and is a co-founder of Aspia Space Ltd., a “NewSpace” company developing novel AI techniques for the downstream analysis of Earth Observation data.
-
Professor Volker Steuber
Steuber is an expert in computational neuroscience (Rothman et al. 2009, Nature 457, 1015) and has served as president of the Organization for Computational Neurosciences (2019-2022).
Volker is Head of the Biocomputation Research Group at the University of Hertfordshire. Research in the Biocomputation Research Group involves the development of computational models to study biological systems, and the application of biologically-inspired machine learning algorithms for the analysis of real-world data. Before joining the university he worked as postdoc at UCL and the University of Antwerp. He received his PhD from the University of Edinburgh and has degrees in artificial intelligence from Edinburgh and in neuroscience from the ETH Zürich. Volker’s current and previous funding includes grants from the Human Frontier Science Program Organization, the German DFG, the BBSRC, Innovate UK and the German National Merit Foundation.
-
Dr Shabnam Kadir
Kadir has pioneered the development of new spike sorting algorithms for electrophysiological experiments which are used by over 300 laboratories worldwide (Rossant, Kadir, et al. 2016 Nature Neuroscience, 19, 634).
Shabnam is a mathematician with a history of interdisciplinary research in computational neuroscience, theoretical physics, pure mathematics and software engineering. Shabnam studied mathematics at Trinity College, Cambridge followed by a DPhil at the Mathematical Institute, Oxford. During her DPhil and subsequent postdoctoral research fellowships at the Fields Institute, Toronto, and the Institute fuer Algebraische Geometrie at Leibniz Universitaet, Hannover, she applied mathematical ideas inspired by string theory to topics in geometry and topology. In particular, she was inspired by the fruitfulness of using computational methods to inspire new and unexpected mathematical conjectures. This led her to start looking at problems in neuroscience during postdoctoral positions at UCL, Imperial, Rutgers and NYU. Her key achievements so far have been in developing machine learning algorithms for the processing and analysis of very large datasets by experimental neuroscientists. She is now a senior lecturer at the University of Hertfordshire and is building new techniques of topological data analysis for use on neuroscientific data, ranging from the auditory system, to vision and olfaction.
-
Professor Michael Schmuker
Schmuker's game-changing research translates processes from biology and the brain into algorithms and solutions for data science, sensory computing, and robotics. He has pioneered brain-derived algorithms for neuromorphic computing (Schmuker et al., PNAS 2014). Michael also frequently collaborates with life scientists and enable data-driven interdisciplinary discovery (Burton et al., eLife 2022; Strutz et al, eLife 2014).
Michael has a degree in Biology, a PhD in Chemistry, and postdoctoral experience in Computational Neuroscience and Computer Science/Engineering. Criss-crossing between these fields, he investigates the sense of smell in animals and transfers the findings into machines. Michael's research involves exploring the nose's remarkable ability to efficiently discriminate between odorants. In a second strand of research, he leads the development of bio-mimetic algorithms to accelerate chemical sensing in robots, with potential applications in agriculture, environmental monitoring, disaster management and security.
-
Dr William Alston
Alston is an expert in signal processing methods and their application to accreting black holes and neutron stars (Alston et al 2020, Nature Astronomy, 4, 597).
Will is an astrophysicist specialising in the application of novel signal processing and machine learning methods to astronomical data. Will obtained his PhD from University of Leicester in 2014 and worked as a postdoctoral research associate at the Institute of Astronomy, Cambridge UK. He then joined the European Space Agency as a Research Fellow, before taking a Senior lecturer position at University of Hertfordshire. His current research is focused on developing algorithms to detect and model signals from black holes, where the goal is to understand gravity in the strong field regime, extreme physics and the role these objects play in shaping the universe. The potential use of these methods across interdisciplinary fields is also explored, for example in modelling the relative growth and health of crops using time-domain multi-spectral Earth Observation imaging.
-
Dr John Evans
Evans conducts research focuses on ideas relating to algebraic topology (Evans 2018, The Quarterly Journal of Mathematics Evans 2021, Communications in Algebra).
John is a mathematician specialising in algebraic topology and algebraic K-theory, with a particular interest in the boundary of topology, geometry and data science. After completing his degree in mathematics from UCL, John undertook a PhD in algebraic topology at UCL under the guidance of Prof. F.E.A. Johnson. There, John developed a specialism in syzygy modules and their interactions under tensor product. Following his PhD, John held several lecturing positions at NUST (China), Coventry University (UK) and University of Reading (UK) before becoming a lecturer in data science and mathematics at the University of Hertfordshire. Over this period, John has developed an interest in the applications of topology, especially to environmental data sets. In this regard, John’s research involves developing and employing techniques arising from topological data analysis which compute topological properties that are stable under perturbations of noisy data.